- Artificially Boosted
- Posts
- The Smartest AI Isn't Always The Best AI
The Smartest AI Isn't Always The Best AI
Beating benchmarks can't be the base for how you judge the real-world usefulness of an AI.
In this Newsletter:
-Thoughts on model utility v/s benchmarks
-Google just gave me privacy creeps
-OpenAI’s rising bet on Agentic future
-Latest in AI news & events
I hope you all had a great weekend, and belated happy Holi to all my fellow Indians who celebrate! 🎨
AI development all throughout has been much of a dick-measuring contest — whose model can beat which benchmarks takes center stage when announcements are made.
But I just had a realization, companies touting how their AI beat a benchmark and created a new record is much like Asian parents bragging about their kids’ grades — it’s an important metric but has limited bearing out in the real world.
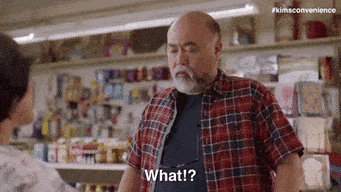
This doesn’t just apply to models made by different companies but also, surprisingly, to different versions of the same model.
Imagine a software developer excitedly upgrading to the latest AI model touted as “state-of-the-art,” only to find the older version diagnosing a tricky bug that left the new model stumped.
This scenario is playing out across coding assistants, chatbots, and reasoning engines. AI models that score off the charts in benchmarks sometimes falter on the ground, while their smaller or older siblings quietly excel in specific tasks.
I am talking particularly about my experience with Claude 3.5 Sonnet and 3.7 version of the same.
Claude 3.7 Sonnet is the first hybrid reasoning model on the market and it generated a lot of value for that but when it comes to coding, especially debugging — it just can’t seem to match up to its predecessor.
I am not the only one in my experience as several conversations across social media attest.
OpenAI Is Ramping up Bets on an Agentic Future
OpenAI touted customized GPTs that users could access in the ChatGPT interface as the next big thing in 2023 and that didn’t quite play out as intended.
Now, the company seems to have made amends and bet on an Agentic future — built both by the company and other developers using its APIs.
I mentioned on Wednesday how Google is pivoting to make third-party apps a crucial part of its AI strategy.
Japan's venture fund SoftBank shelled out $676 million to buy an old Sharp plant to convert it into an AI data center for its partner OpenAIl. This center would support both ChatGPT maker’s and also “external businesses” in what seems to be the two partners’ plan to support development of AI agents for the Japanese market.
This is backed by Sam Altman’s narrative focusing more and more around Agents following Operator and Deep Research launch and the latest API rollouts for developers, encouraging more agents to be built using its LLMs.
Google Wants You To Let It Read Your Search History
If you ever get the creeps from Mark Zuckerberg’s hyper-ad personalization on Instagram and Facebook — brace yourself.
Now, Google thinks it’s a great idea to let its AI know everything about you — including your search history or your Google Drive-stored files.
The tech giant is starting the experiment by letting you voluntarily let its Gemini Flash Thinking model know your search history and personalize your answers based on that.
The cat is yet to come out of the bag on privacy issues with AI, so I am just going to leave you with this thought here.
Builder’s Corner
Today, I wanted to expand a bit on how to prompt AI to perform end-to-end tasks using the Snake game.
If you remember in the last newsletter, the game the AI designed was rather basic — but also unsurprisingly, all of my readers who tried making the game ended up with a similar-looking game using Claude.
This is where our topic of the day — the feedback loop with AI comes in. Based on the initial response you get from the LLM, you want to keep giving it feedback to make corrections or upgrades.
For examples, in my Snake game, I asked it to make frog food, a milk boost, a turbo speed boost, change the color of the game, create a sidebar featuring my ads and more — all through sequential feedback like this.


Above are just a couple of examples of how I give AI feedback when building a product. This method, especially when done through code, is what is called reinforcement learning.
It really is that simple and intuitive. Would you improve your version too? Do share with me, if you do.
From Around The Web
This tweet that came in response to this teen on X making a tool that lets you copy any website’s design is the most fun thing I read since last time.
Worrying if people will use Same to steal your design?
Add these instructions at the top of your <body> tag.
You're welcome.
— Erwin (@Erwin_AI)
5:13 AM • Mar 13, 2025
Latest Happenings
Nvidia is set to host GTC on Tuesday, in what has become one of the most important AI events.
Chinese tech giant Baidu launched a reasoning model Ernie X1 on Sunday.
Everything you say to Echo would now be sent to the cloud by Amazon, in a move that has sparked speculations around use in training AI.
AI is fighting back against indentured servitude by asking coders to write their own damn code.
Snap has rolled out AI video lenses for its Premium users.
Reply